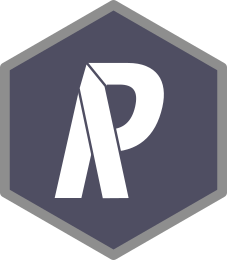
Evaluate connectivity of solution
Source:R/eval_connectivity_summary.R
eval_connectivity_summary.Rd
Calculate the connectivity held within a solution to a conservation planning problem. This summary statistic evaluates the connectivity of a solution using pair-wise connectivity values between combinations of planning units. It is specifically designed for symmetric connectivity data.
Usage
# S4 method for ConservationProblem,ANY,ANY,matrix
eval_connectivity_summary(x, solution, zones, data)
# S4 method for ConservationProblem,ANY,ANY,Matrix
eval_connectivity_summary(x, solution, zones, data)
# S4 method for ConservationProblem,ANY,ANY,data.frame
eval_connectivity_summary(x, solution, zones, data)
# S4 method for ConservationProblem,ANY,ANY,dgCMatrix
eval_connectivity_summary(x, solution, zones, data)
# S4 method for ConservationProblem,ANY,ANY,array
eval_connectivity_summary(x, solution, zones, data)
Arguments
- x
problem()
object.- solution
numeric
,matrix
,data.frame
,terra::rast()
, orsf::sf()
object. The argument should be in the same format as the planning unit cost data in the argument tox
. See the Solution format section for more information.- zones
matrix
orMatrix
object describing the level of connectivity between different zones. Each row and column corresponds to a different zone in the argument tox
, and cell values indicate the level of connectivity between each combination of zones. Cell values along the diagonal of the matrix represent the level of connectivity between planning units allocated to the same zone. Cell values must lay between 1 and -1, where negative values favor solutions with weak connectivity. The default argument tozones
is an identity matrix (i.e., a matrix with ones along the matrix diagonal and zeros elsewhere), so that planning units are only considered to be connected when they are allocated to the same zone. This argument is required when working with multiple zones and the argument todata
is amatrix
orMatrix
object. If the argument todata
is anarray
ordata.frame
with data for multiple zones (e.g., using the"zone1"
and"zone2"
column names), this argument must explicitly be set toNULL
otherwise an error will be thrown.- data
matrix
,Matrix
,data.frame
, orarray
object containing connectivity data. The connectivity values correspond to the strength of connectivity between different planning units. Thus connections between planning units that are associated with higher values are more favorable in the solution. See the Data format section for more information.
Value
A tibble::tibble()
object describing the connectivity of the
solution.
It contains the following columns:
- summary
character
description of the summary statistic. The statistic associated with the"overall"
value in this column is calculated using the entire solution (including all management zones if there are multiple zones). If multiple management zones are present, then summary statistics are also provided for each zone separately (indicated using zone names).- connectivity
numeric
connectivity value. Greater values correspond to solutions associated with greater connectivity. Thus conservation planning exercises typically prefer solutions with greater values.
Details
This summary statistic is comparable to the Connectivity_In
metric
reported by the
Marxan software (Ball et al. 2009).
It is calculated using the same equations used to penalize solutions
with connectivity data (i.e., add_connectivity_penalties()
).
Specifically, it is calculated as the sum of the pair-wise connectivity
values in the argument to data
, weighted by the value of the planning
units in the solution.
Solution format
Broadly speaking, the argument to solution
must be in the same format as
the planning unit data in the argument to x
.
Further details on the correct format are listed separately
for each of the different planning unit data formats:
x
hasnumeric
planning unitsThe argument to
solution
must be anumeric
vector with each element corresponding to a different planning unit. It should have the same number of planning units as those in the argument tox
. Additionally, any planning units missing cost (NA
) values should also have missing (NA
) values in the argument tosolution
.x
hasmatrix
planning unitsThe argument to
solution
must be amatrix
vector with each row corresponding to a different planning unit, and each column correspond to a different management zone. It should have the same number of planning units and zones as those in the argument tox
. Additionally, any planning units missing cost (NA
) values for a particular zone should also have a missing (NA
) values in the argument tosolution
.x
hasterra::rast()
planning unitsThe argument to
solution
be aterra::rast()
object where different cells correspond to different planning units and layers correspond to a different management zones. It should have the same dimensionality (rows, columns, layers), resolution, extent, and coordinate reference system as the planning units in the argument tox
. Additionally, any planning units missing cost (NA
) values for a particular zone should also have missing (NA
) values in the argument tosolution
.x
hasdata.frame
planning unitsThe argument to
solution
must be adata.frame
with each column corresponding to a different zone, each row corresponding to a different planning unit, and cell values corresponding to the solution value. This means that if adata.frame
object containing the solution also contains additional columns, then these columns will need to be subsetted prior to using this function (see below for example withsf::sf()
data). Additionally, any planning units missing cost (NA
) values for a particular zone should also have missing (NA
) values in the argument tosolution
.x
hassf::sf()
planning unitsThe argument to
solution
must be asf::sf()
object with each column corresponding to a different zone, each row corresponding to a different planning unit, and cell values corresponding to the solution value. This means that if thesf::sf()
object containing the solution also contains additional columns, then these columns will need to be subsetted prior to using this function (see below for example). Additionally, the argument tosolution
must also have the same coordinate reference system as the planning unit data. Furthermore, any planning units missing cost (NA
) values for a particular zone should also have missing (NA
) values in the argument tosolution
.
Data format
The argument to data
can be specified using several different formats.
data
as amatrix
/Matrix
objectwhere rows and columns represent different planning units and the value of each cell represents the strength of connectivity between two different planning units. Cells that occur along the matrix diagonal are treated as weights which indicate that planning units are more desirable in the solution. The argument to
zones
can be used to control the strength of connectivity between planning units in different zones. The default argument forzones
is to treat planning units allocated to different zones as having zero connectivity.data
as adata.frame
objectcontaining columns that are named
"id1"
,"id2"
, and"boundary"
. Here, each row denotes the connectivity between a pair of planning units (per values in the"id1"
and"id2"
columns) following the Marxan format. If the argument tox
contains multiple zones, then the"zone1"
and"zone2"
columns can optionally be provided to manually specify the connectivity values between planning units when they are allocated to specific zones. If the"zone1"
and"zone2"
columns are present, then the argument tozones
must beNULL
.data
as anarray
objectcontaining four-dimensions where cell values indicate the strength of connectivity between planning units when they are assigned to specific management zones. The first two dimensions (i.e., rows and columns) indicate the strength of connectivity between different planning units and the second two dimensions indicate the different management zones. Thus the
data[1, 2, 3, 4]
indicates the strength of connectivity between planning unit 1 and planning unit 2 when planning unit 1 is assigned to zone 3 and planning unit 2 is assigned to zone 4.
References
Ball IR, Possingham HP, and Watts M (2009) Marxan and relatives: Software for spatial conservation prioritisation in Spatial conservation prioritisation: Quantitative methods and computational tools. Eds Moilanen A, Wilson KA, and Possingham HP. Oxford University Press, Oxford, UK.
See also
See summaries for an overview of all functions for summarizing solutions.
Also, see add_connectivity_penalties()
to penalize solutions with low
connectivity.
Other summaries:
eval_asym_connectivity_summary()
,
eval_boundary_summary()
,
eval_cost_summary()
,
eval_feature_representation_summary()
,
eval_n_summary()
,
eval_target_coverage_summary()
Examples
# \dontrun{
# set seed for reproducibility
set.seed(500)
# load data
sim_pu_raster <- get_sim_pu_raster()
sim_features <- get_sim_features()
sim_zones_pu_polygons <- get_sim_zones_pu_polygons()
sim_zones_features <- get_sim_zones_features()
# build minimal conservation problem with raster data
p1 <-
problem(sim_pu_raster, sim_features) %>%
add_min_set_objective() %>%
add_relative_targets(0.1) %>%
add_binary_decisions() %>%
add_default_solver(verbose = FALSE)
# solve the problem
s1 <- solve(p1)
# print solution
print(s1)
#> class : SpatRaster
#> dimensions : 10, 10, 1 (nrow, ncol, nlyr)
#> resolution : 0.1, 0.1 (x, y)
#> extent : 0, 1, 0, 1 (xmin, xmax, ymin, ymax)
#> coord. ref. : Undefined Cartesian SRS
#> source(s) : memory
#> varname : sim_pu_raster
#> name : layer
#> min value : 0
#> max value : 1
# plot solution
plot(s1, main = "solution", axes = FALSE)
# simulate a connectivity matrix to describe the relative strength
# of connectivity between different planning units
# for brevity, we will use cost data here so that pairs
# of adjacent planning units with higher cost values will have a
# higher connectivity value
# (but see ?connectivity_matrix for more information)
cm1 <- connectivity_matrix(sim_pu_raster, sim_pu_raster)
# calculate connectivity associated with the solution
r1 <- eval_connectivity_summary(p1, s1, data = cm1)
print(r1)
#> # A tibble: 1 × 2
#> summary connectivity
#> <chr> <dbl>
#> 1 overall 198.
# build multi-zone conservation problem with polygon data
p2 <-
problem(
sim_zones_pu_polygons, sim_zones_features,
cost_column = c("cost_1", "cost_2", "cost_3")
) %>%
add_min_set_objective() %>%
add_relative_targets(matrix(runif(15, 0.1, 0.2), nrow = 5, ncol = 3)) %>%
add_binary_decisions() %>%
add_default_solver(verbose = FALSE)
# solve the problem
s2 <- solve(p2)
# print solution
print(s2)
#> Simple feature collection with 90 features and 9 fields
#> Geometry type: POLYGON
#> Dimension: XY
#> Bounding box: xmin: 0 ymin: 0 xmax: 1 ymax: 1
#> Projected CRS: Undefined Cartesian SRS
#> # A tibble: 90 × 10
#> cost_1 cost_2 cost_3 locked_1 locked_2 locked_3 solution_1_zone_1
#> <dbl> <dbl> <dbl> <lgl> <lgl> <lgl> <dbl>
#> 1 216. 183. 205. FALSE FALSE FALSE 0
#> 2 213. 189. 210. FALSE FALSE FALSE 0
#> 3 207. 194. 215. TRUE FALSE FALSE 0
#> 4 209. 198. 219. FALSE FALSE FALSE 0
#> 5 214. 200. 221. FALSE FALSE FALSE 0
#> 6 214. 203. 225. FALSE FALSE FALSE 0
#> 7 211. 209. 223. FALSE FALSE FALSE 0
#> 8 210. 212. 222. TRUE FALSE FALSE 0
#> 9 204. 218. 214. FALSE FALSE FALSE 0
#> 10 213. 183. 206. FALSE FALSE FALSE 0
#> # ℹ 80 more rows
#> # ℹ 3 more variables: solution_1_zone_2 <dbl>, solution_1_zone_3 <dbl>,
#> # geom <POLYGON [m]>
# create new column representing the zone id that each planning unit
# was allocated to in the solution
s2$solution <- category_vector(
s2[, c("solution_1_zone_1", "solution_1_zone_2", "solution_1_zone_3")]
)
s2$solution <- factor(s2$solution)
# plot solution
plot(s2[, "solution"])
# simulate connectivity matrix
# here, we will add a new column to sim_zones_pu_polygons with
# randomly simulated values and create a connectivity matrix
# based on the average simulated values of adjacent planning units
sim_zones_pu_polygons$con <- runif(nrow(sim_zones_pu_polygons))
cm2 <- connectivity_matrix(sim_zones_pu_polygons, "con")
# calculate connectivity associated with the solution
r2 <- eval_connectivity_summary(
p2, s2[, c("solution_1_zone_1", "solution_1_zone_2", "solution_1_zone_3")],
data = cm2
)
print(r2)
#> # A tibble: 4 × 2
#> summary connectivity
#> <chr> <dbl>
#> 1 overall 4.04
#> 2 zone_1 1.92
#> 3 zone_2 1.40
#> 4 zone_3 0.722
# }